AI Conference
International Conference for AI-based Assessments in STEM Education
The Framework for K-12 Science Education has set forth an ambitious vision for science learning by integrating disciplinary science ideas, scientific and engineering practices, and crosscutting concepts, so that students could develop competence to meet the STEM challenges of the 21st century. Achieving this vision requires transformation of assessment practices from relying on multiple-choice items to performance-based knowledge-in-use tasks. However, these performance-based constructed-response items often prohibit timely feedback, which, in turn, has hindered science teachers from using these assessments. Artificial Intelligence (AI) has demonstrated great potential to meet this assessment challenge. To tackle this challenge, experts in assessment, AI, and science education will gather for a two-day conference at University of Georgia to generate knowledge of integrating AI in science assessment.
National Science Foundation
Funding: $60,000
Office of the Senior Vice President for Academic Affairs and Provost
Funding: $5,000
Timeline: 2021-2022
Project Members

Xiaoming Zhai
Principal Investigator
University of Georgia
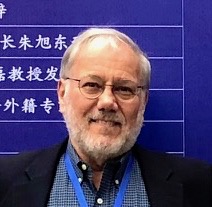
Joseph Krajcik
Co-Principal Investigator
Michigan State University
Keynote Speakers
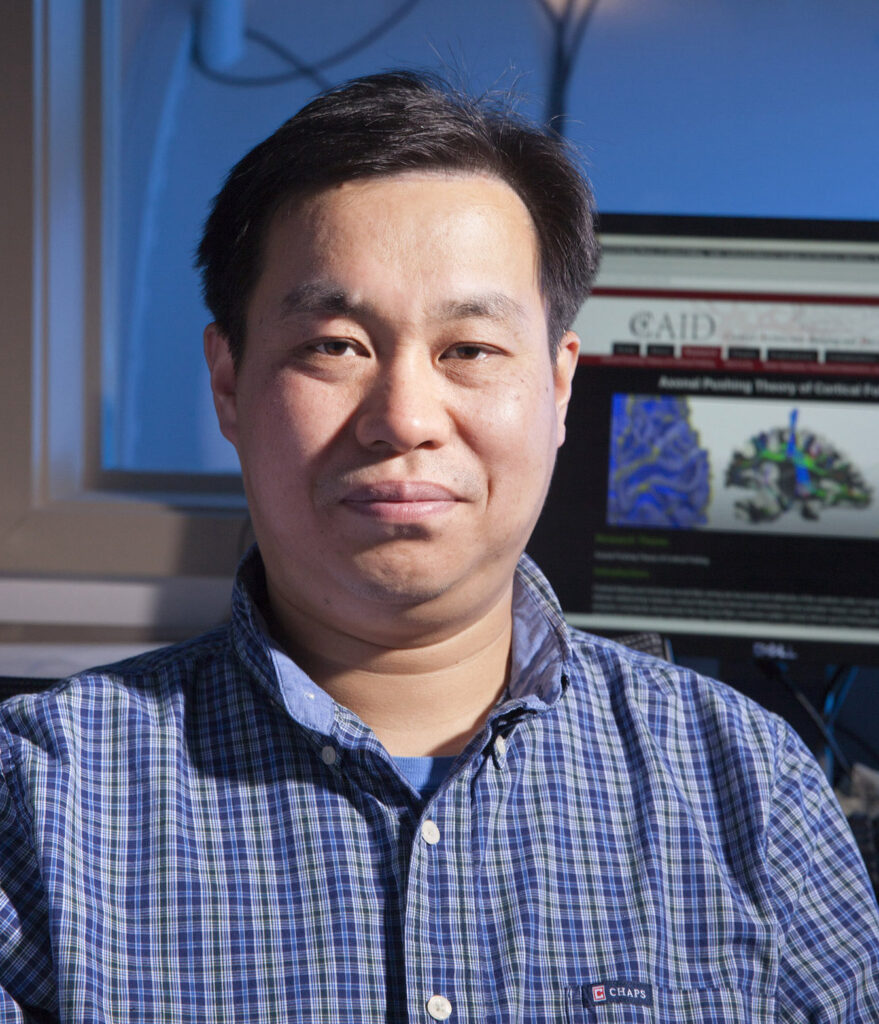
Tianming Liu
University of Georgia
Title: AI Research and Education: A Whole-society Approach
Abstract: In this talk, the speaker will share his experience and vision in AI research, AI education, and the role of AI in STEM education. The speaker will propose a new concept of a whole-society approach to AI literacy, AI education and AI-empowered society.
Biography: Dr. Tianming Liu is a Distinguished Research Professor (since 2017) and a Full Professor of Computer Science (since 2015) at the University of Georgia (UGA). Dr. Liu is also an affiliated faculty (by courtesy) with UGA Bioimaging Research Center (BIRC), UGA Institute of Bioinformatics (IOB), UGA Neuroscience Ph.D. Program, and UGA Institute of Artificial Intelligence (IAI). Dr. Liu’s primary research interests are brain imaging, computational neuroscience, and brain-inspired artificial intelligence, and he has published over 370 papers in these areas. Dr. Liu is the recipient of the NIH Career Award (2007-2012) and the NSF CAREER Award (2012-2017). Dr. Liu is a Fellow of AIMBE (inducted in 2018) and was the General Chair of MICCAI 2019.
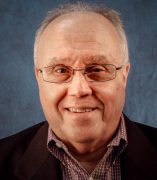
James Pellegrino
University of Illinois Chicago
Title: A New Era of STEM Assessment: Technology and AI
Abstract: This talk will discuss what technology and AI makes possible in the new era of science assessment, why it is needed, and some of the challenges with bringing this vision to fruition. For purposes of framing the discussion, the talk will reference the three elements of the Assessment Triangle — Cognition, Observation and Interpretation — that collectively describe assessment as a process of reasoning from evidence (Pellegrino, Chudowsky, & Glaser, 2001).
Biography: James Pellegrino is a Liberal Arts and Sciences Distinguished Professor and Distinguished Professor of Education at the University of Illinois at Chicago (UIC). He is a founding Co-director of UIC’s interdisciplinary Learning Sciences Research Institute. Pellegrino’s research and development interests focus on children’s and adults’ thinking and learning and the implications of cognitive research and theory for assessment and instructional practice. Much of his current work is focused on analyses of complex learning and instructional environments, including those incorporating powerful information technology tools, with the goal of better understanding the nature of student learning and the conditions that enhance deep understanding. A special concern of his research is the incorporation of effective formative assessment practices, assisted by technology, to maximize student learning and understanding. Increasingly his research and writing have focused on the role of cognitive theory and technology in educational reform and translating results from the educational and psychological research arenas into implications for practitioners and policymakers. He has led NRC study committees including the Committee that issued Knowing What Students Know. He was a member of the Committee on A Framework for K-12 Science Education and Co-chaired the Committee on Developing Assessments of Science Proficiency in K-12. He is involved in work to advance the validity of machine learning-based assessments.

Marcia C. Linn
UC Berkeley
Title: AI Based Assessments: Design, Validity, and Impact
Abstract: We are making progress in developing valid AI-based assessments. What next? What new designs for assessment are enabled by AI-based assessments? How can we design AI-based assessments and instruction to fully realize the potential of these advances? This talk will illustrate possibilities for assessment design and opportunities for synergies between AI-based assessments and classroom instruction in STEM.
Biography: Marcia C. Linn is the Evelyn Lois Corey Professor of Instructional Science at the Berkeley School of Education, University of California, Berkeley. She specializes in science and technology. She is a member of the National Academy of Education and a Fellow of the American Association for the Advancement of Science (AAAS), the American Psychological Association (APA), the Association for Psychological Science (APS), the American Educational Research Association (AERA), and the International Society of the Learning Sciences (ISLS). She has served as President of the International Society of the Learning Sciences (ISLS), Chair of the AAAS Education Section, and on the boards of the AAAS, the Educational Testing Service Graduate Record Examination, the McDonnell Foundation Cognitive Studies in Education Practice, and the National Science Foundation Education and Human Resources Directorate. Awards include the National Association for Research in Science Teaching Award for Lifelong Distinguished Contributions to Science Education, the American Educational Research Association Willystine Goodsell Award, and the Council of Scientific Society Presidents first award for Excellence in Educational Research. Linn earned her Ph. D. at Stanford University where she worked with Lee Cronbach. She spent a year in Geneva working with Jean Piaget, a year in Israel as a Fulbright Professor, and a year in London at University College. She has been a fellow at the Center for Advanced Study in Behavioral Sciences three times and a Bellagio Center Writing Resident twice. Her books include Computers, Teachers, Peers (2000), Internet Environments for Science Education (2004), Designing Coherent Science Education (2008), WISE Science (2009), and Science Teaching and Learning: Taking Advantage of Technology to Promote Knowledge Integration (2011) [Chinese Translation, 2015]. She chairs the Technology, Education—Connections (TEC) series for Teachers College Press.
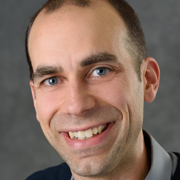
Kevin Haudek
Michigan State University
Title: Exploring Attributes of Successful Machine Learning Assessments for Scoring of Undergraduate Constructed Responses
Abstract: The use of Machine Learning (ML) to automatically score text-based constructed responses (CRs) has increased the use of authentic assessments in STEM by reducing the burden of evaluation and feedback on instructors. However, one limitation is the difficulty of creating well-performing assessments, as they typically require multiple rounds of item and model development. This results in a relatively small number of assessments and heavily limits the item contexts that can be presented. As current frameworks (NRC, 2012; AAAS, 2011) have called for students to learn and apply core concepts and skills across different contexts in STEM, the limited items hinder properly assessing students. Unfortunately, little is known about what allows for easy creation of the associated ML model. Instead, development relies on iterative cycles of trial and error, increasing the amount of time needed for development. Over the last several years, our group has developed over 50 different items and associated ML models that predict student responses based on validated learning progressions in undergraduate science. These items span five key concepts in the field of physiology: Bulk Flow, Diffusion, Ion Flow, Mass Balance, and Water Movement. As model development for these items have had varying success, we propose examining this extensive set to explore attributes of the items that allow for better model development.
Biography: Kevin Haudek is an assistant professor in the CREATE for STEM Institute and Biochemistry and Molecular Biology department at Michigan State University. He engages in discipline-based education research (DBER). His research interests focus on uncovering undergraduate’s thinking about key concepts in biology and scientific practices using formative assessments. As part of this work, he investigates the application of machine learning and natural language processing to help evaluate student writing. These tools enable both exploration of ideas in student writing, as well as the ability to predict classifications for short, content-rich responses. He is currently leading projects associated with the Automated Analysis of Constructed Response (AACR) group, which conducts research of such tools and assessments.
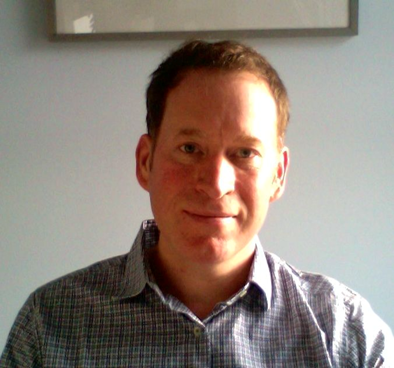
Ross H. Nehm
Stony Brook University
Title: AI in Biology Education: Automation and Transformation
Abstract: Over the past several decades, biology education research has increasingly leveraged Artificial Intelligence (AI) tools (e.g., data mining, machine learning) to explore a range of topics in assessment, cognition, and learning. This talk will argue that recent critical reviews and metanalyses of AI contributions to science education have fallen prey to presentism; consequently, these studies have mischaracterized the impact of AI on the field. Employing a more expansive analytical frame, I will analyze the historical successes, failures, and future challenges of AI in biology education research and practice. I will end by discussing the unique aspects of biological epistemology that will need to be considered when using AI to assess and understand knowledge in practice (e.g., three-dimensional learning) within and beyond the life sciences.
Biography: Ross Nehm is PI of the Biology Education Research Lab, and Professor in the department of ecology and evolution and the program in science education at Stony Brook University (SUNY). His lab was an early pioneer in the use of AI in studies of biology learning and assessment, and it continues to advance understanding of its potential to improve learning outcomes in undergraduate settings. Dr. Nehm completed his graduate work in biology and science education at the University of California-Berkeley and Columbia University. His major awards include an NSF CAREER award, a student mentoring award from CUNY, and a teaching award from Berkeley. He was named an Education Fellow in the Life Sciences by the U.S. National Academies and has served in academic leadership roles including as Editor-in-Chief of the journal Evolution: Evolution Education and Outreach, Associate Editor of Science & Education, Associate Editor of the Journal of Research in Science Teaching, Editor of CBE-Life Sciences Education, and a board member of several other journals. He has served on the research advisory boards of numerous federally funded science education projects, the National Science Foundation’s Committee of Visitors, and many NSF panels as Chair. His research findings have been featured in The New Republic, Science magazine’s Editor’s Choice, CBS News, and many other outlets.
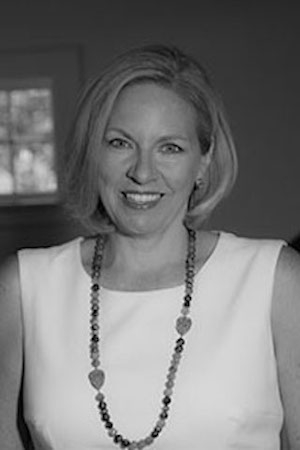
Janice Gobert
Rutgers Graduate School of Education
Title: AI in Science Inquiry
Abstract: The main goal of this presentation is to describe how rigorous assessment design frameworks and new computational techniques can be used to design and develop assessments in order to realize the reform envisioned in frameworks for 21st century skills including the Next Generation Science Standards (2013). The presentation will describe Inq-ITS (Inquiry-Intelligent Tutoring) and its accompanying teacher dashboard Inq-Blotter, which are technology-based systems designed expressly to assess and support students’ competencies at science practices and support teachers’ pedagogical practice related to these practices. To do so, we will provide an overview of the design, data-collection, and data-analysis efforts for Inq-ITS. We will also describe how we used key computational techniques from knowledge-engineering, educational data mining, and natural language processing in order to analyze data from students’ log files and open-responses in this environment. These algorithms are used to automatically score students’ inquiry skills and scaffold them via our digital agent Rex on the practices in real time as they engage in inquiry, as well as provide teachers with fine-grained formative assessment data, alerts, and TIPS (Teacher Inquiry Practice Supports) to support real time instruction of the science practices.
Biography: Janice Gobert, Ph.D. (Cognitive Science, University of Toronto). Gobert is a Learning Scientist with 25+ years’ experience. She is a Professor of Educational Psychology and Learning Sciences at Rutgers Graduate School of Education, and Faculty in the Maker Space Certificate for teachers. She has successfully executed many STEM projects (~25 M to date). She has extensive expertise in model-based learning and assessment, the design of technology-based materials, and the analyses of quantitative data (log data, performance and classical assessment data) and qualitative data (think aloud data, students’ explanations, models/drawings). She was the N. American Editor of the International Journal of Science Education (2000-2006). Janice is the Founding CEO of Apprendis, LLC, which is an ed tech startup productizing Inq-ITS and Inq-Blotter (inqits.com). She is the lead visionary on Inq-ITS. Gobert is also lead inventor on 3 algorithm patents used in Inq-ITS and Inq-Blotter, and she also has additional patents on eye-tracking.